Deliver safe, reliable and predictable LLM results
As companies increasingly leverage generative AI, maintaining model consistency becomes crucial. Ensuring that AI models deliver reliable and uniform results across different applications fortifies trust and enhances user experience.
How we can help
While it is often difficult to manage the inherent probabilistic nature of LLMs, our teams have deep experience applying a variety of foundational techniques to manage around this inconsistency and increase model performance in an effort to reduce latency, improve accuracy, and reduce costs.
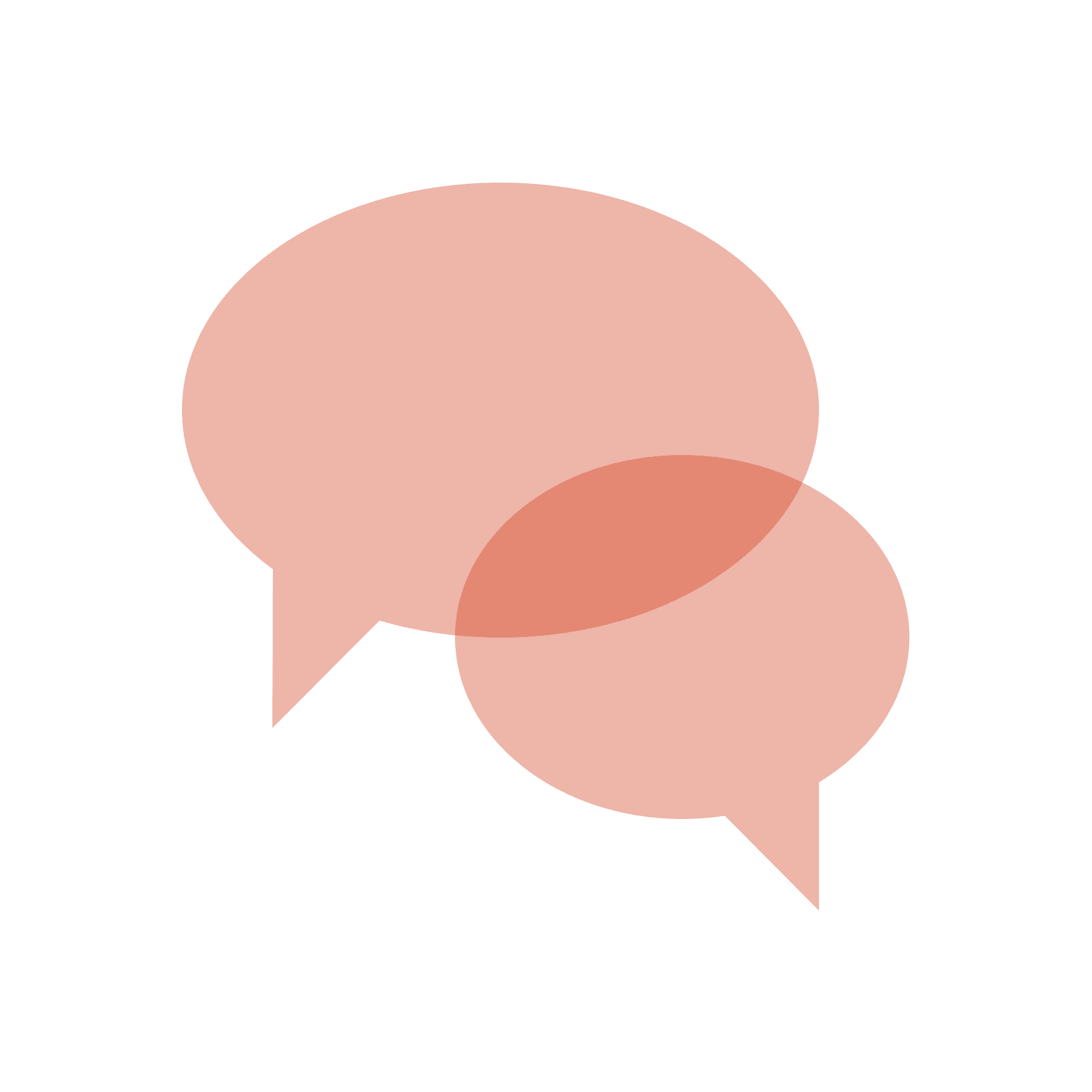
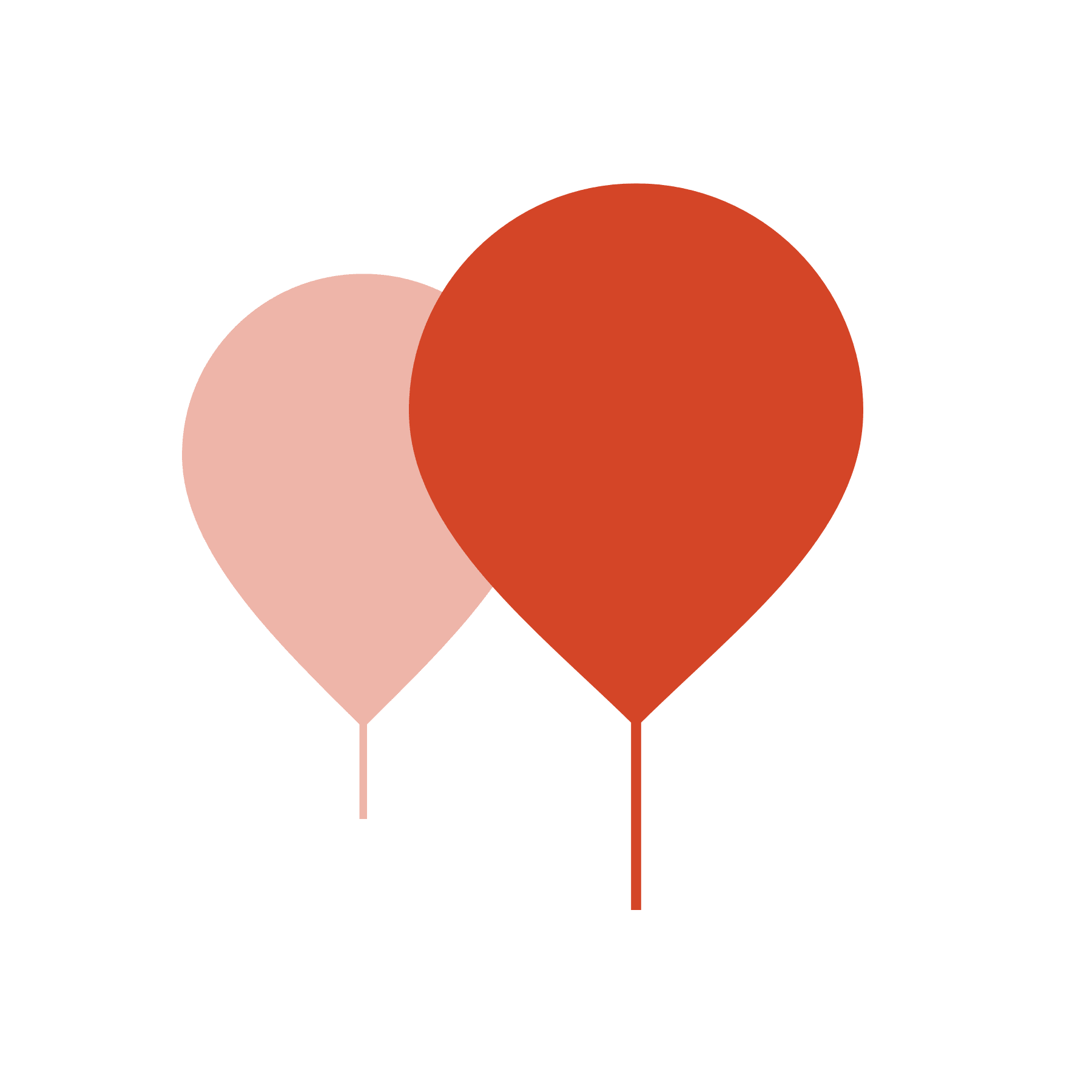
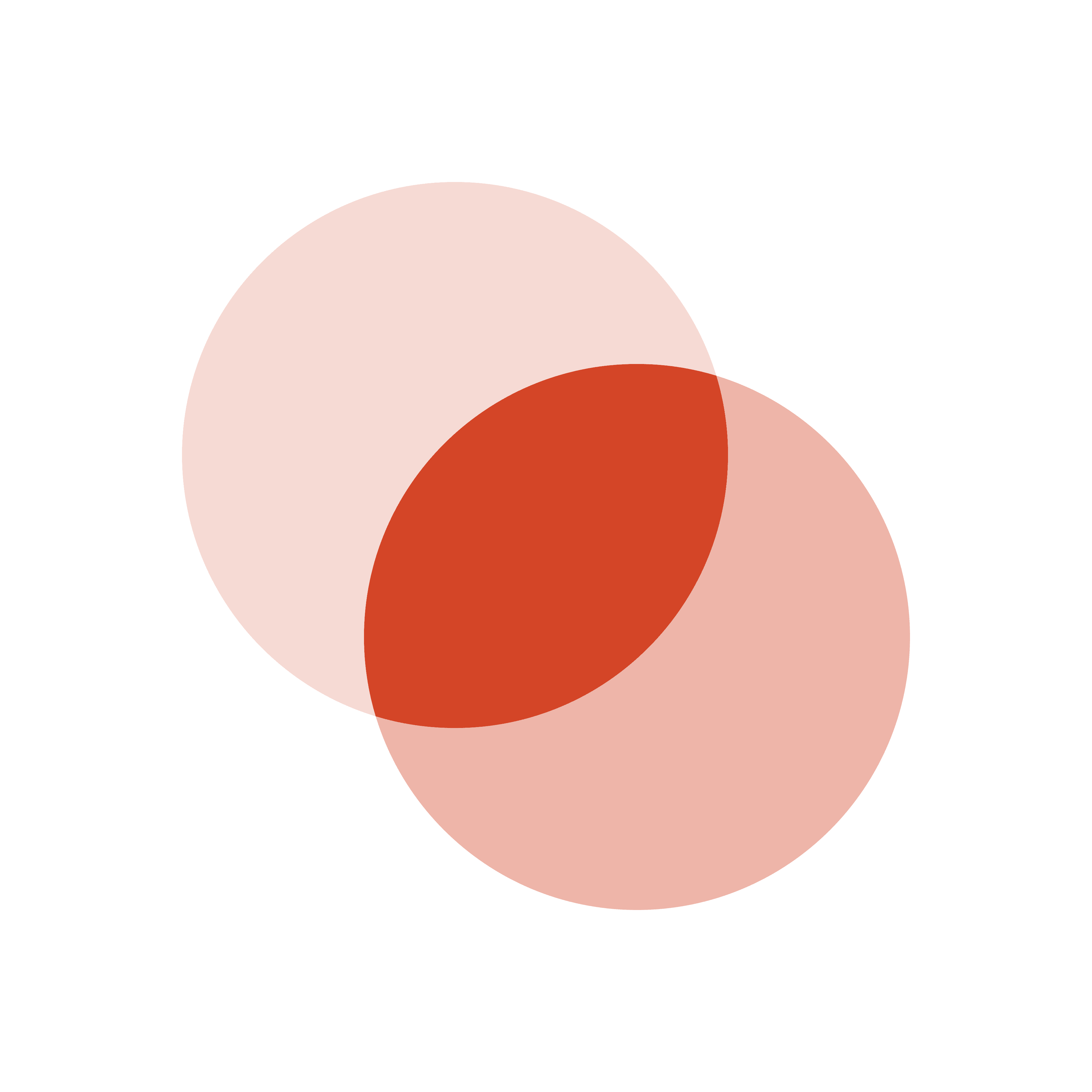
Optimize the prompt
Ground the model
Constrain model behaviour
Our team employs advanced prompt engineering techniques, including few-shot learning, to effectively guide the model towards generating more accurate and consistent responses. By refining the input format and context, we help improve overall model performance while reducing latency and costs.
To mitigate the risk of "hallucinated" information, we implement retrieval-augmented generation (RAG) techniques that ground the model's output in relevant, factual data. Our approaches include simple retrieval, embedding formatting, metadata filtering, contextual retrieval, cross-encoder reranking, HyDE retrieval, chain of thought reasoning, auto-evaluation, and self-consistency.
We offer customization options to ensure your model behaves as expected, such as JSON mode for structured outputs, reproducible outputs using a seed for consistency, and fine-tuning to adapt the model's performance to specific domain knowledge or use cases. By tailoring the model's behaviour, we help you achieve reliable and predictable LLM results.
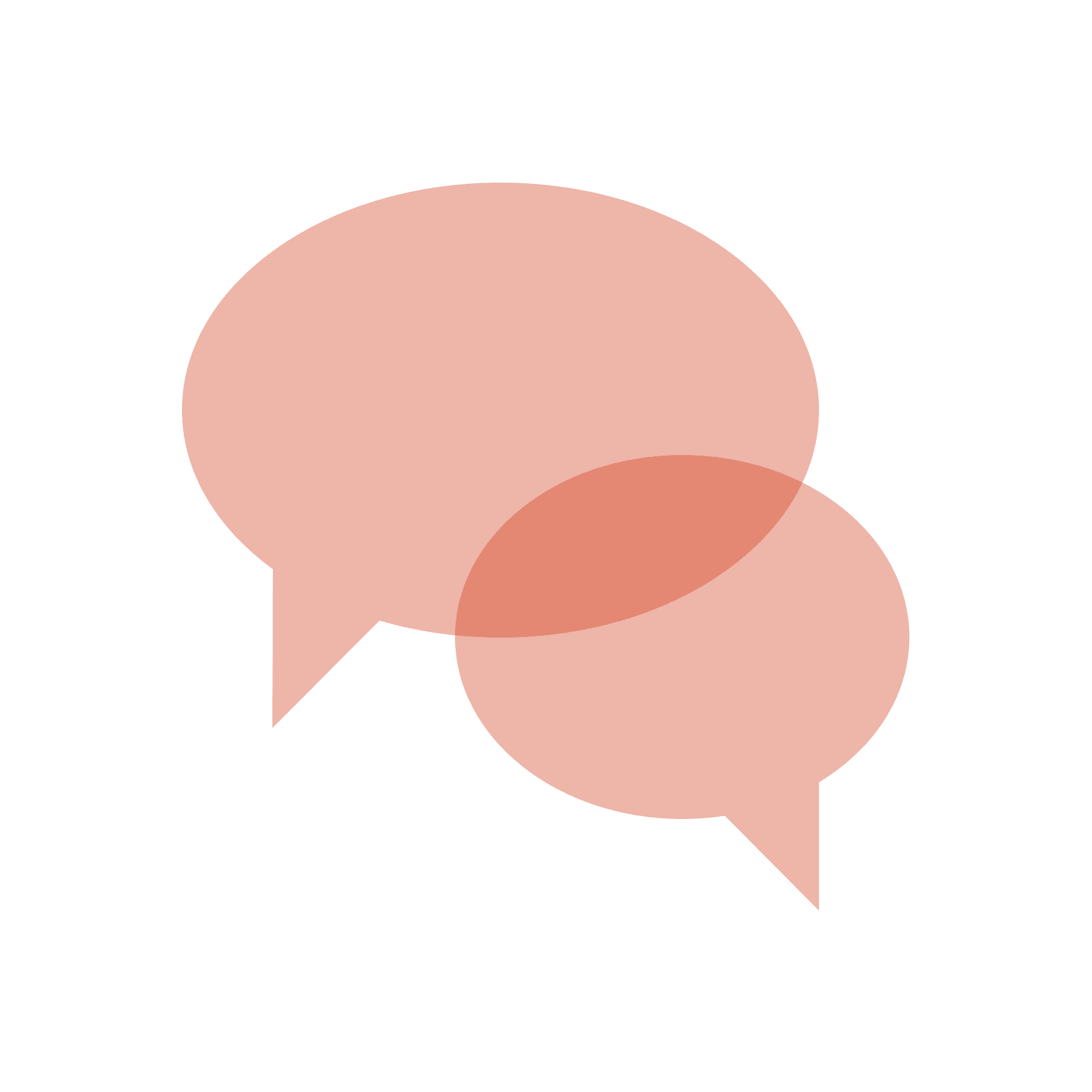
Optimize the prompt
Our team employs advanced prompt engineering techniques, including few-shot learning, to effectively guide the model towards generating more accurate and consistent responses. By refining the input format and context, we help improve overall model performance while reducing latency and costs.
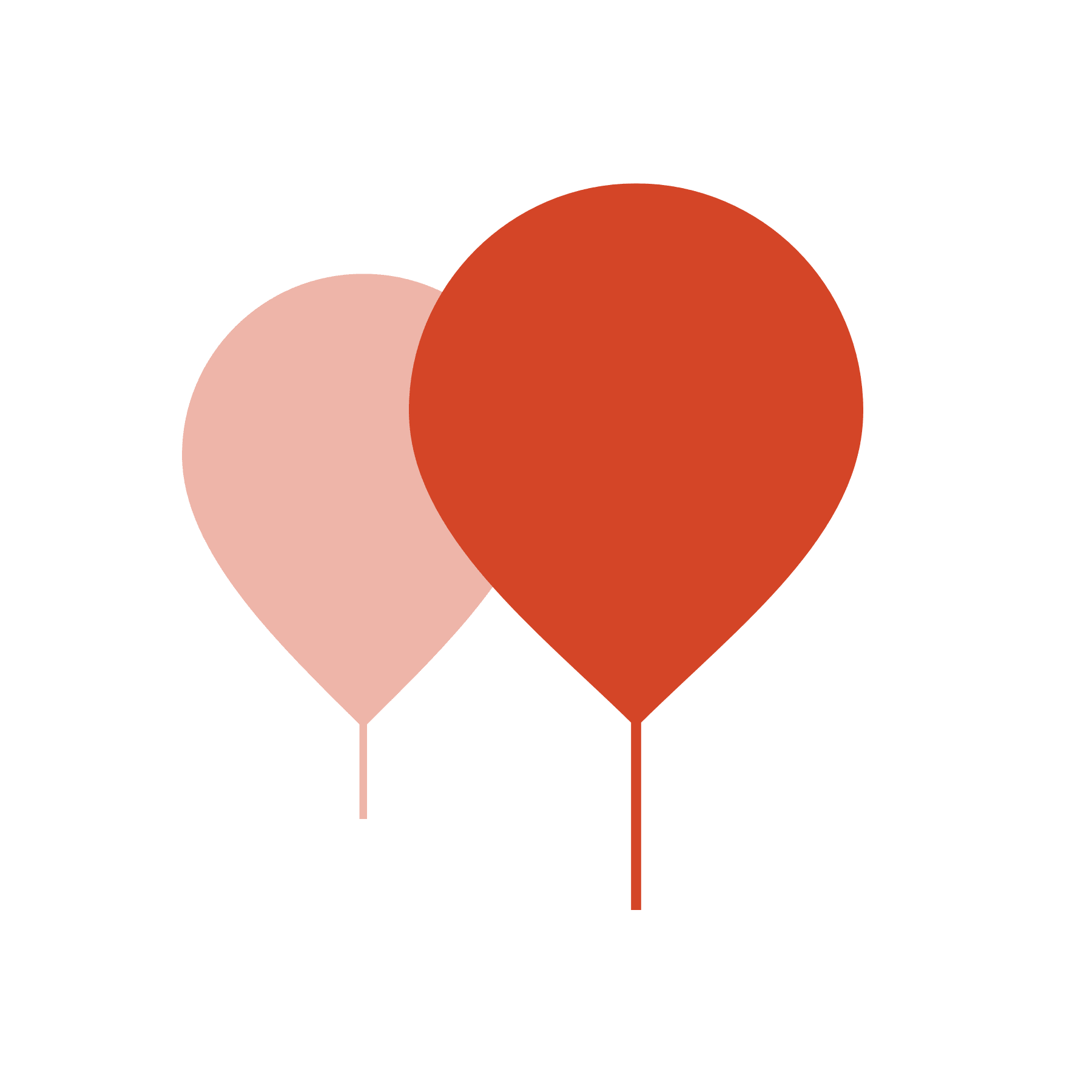
Ground the model
To mitigate the risk of "hallucinated" information, we implement retrieval-augmented generation (RAG) techniques that ground the model's output in relevant, factual data. Our approaches include simple retrieval, embedding formatting, metadata filtering, contextual retrieval, cross-encoder reranking, HyDE retrieval, chain of thought reasoning, auto-evaluation, and self-consistency.
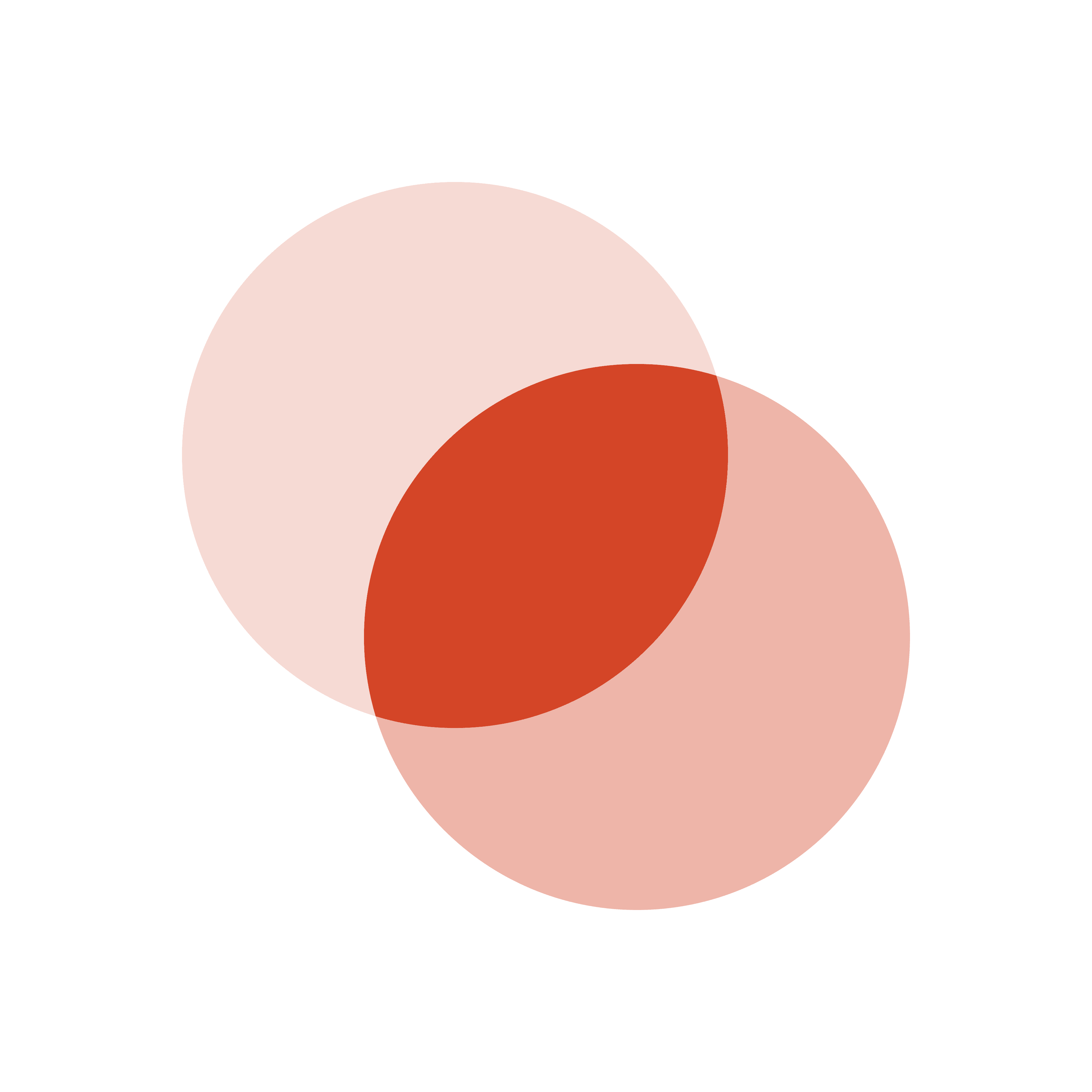
Constrain model behaviour
We offer customization options to ensure your model behaves as expected, such as JSON mode for structured outputs, reproducible outputs using a seed for consistency, and fine-tuning to adapt the model's performance to specific domain knowledge or use cases. By tailoring the model's behaviour, we help you achieve reliable and predictable LLM results.
Companies we've helped
New Product Development & Artificial Intelligence
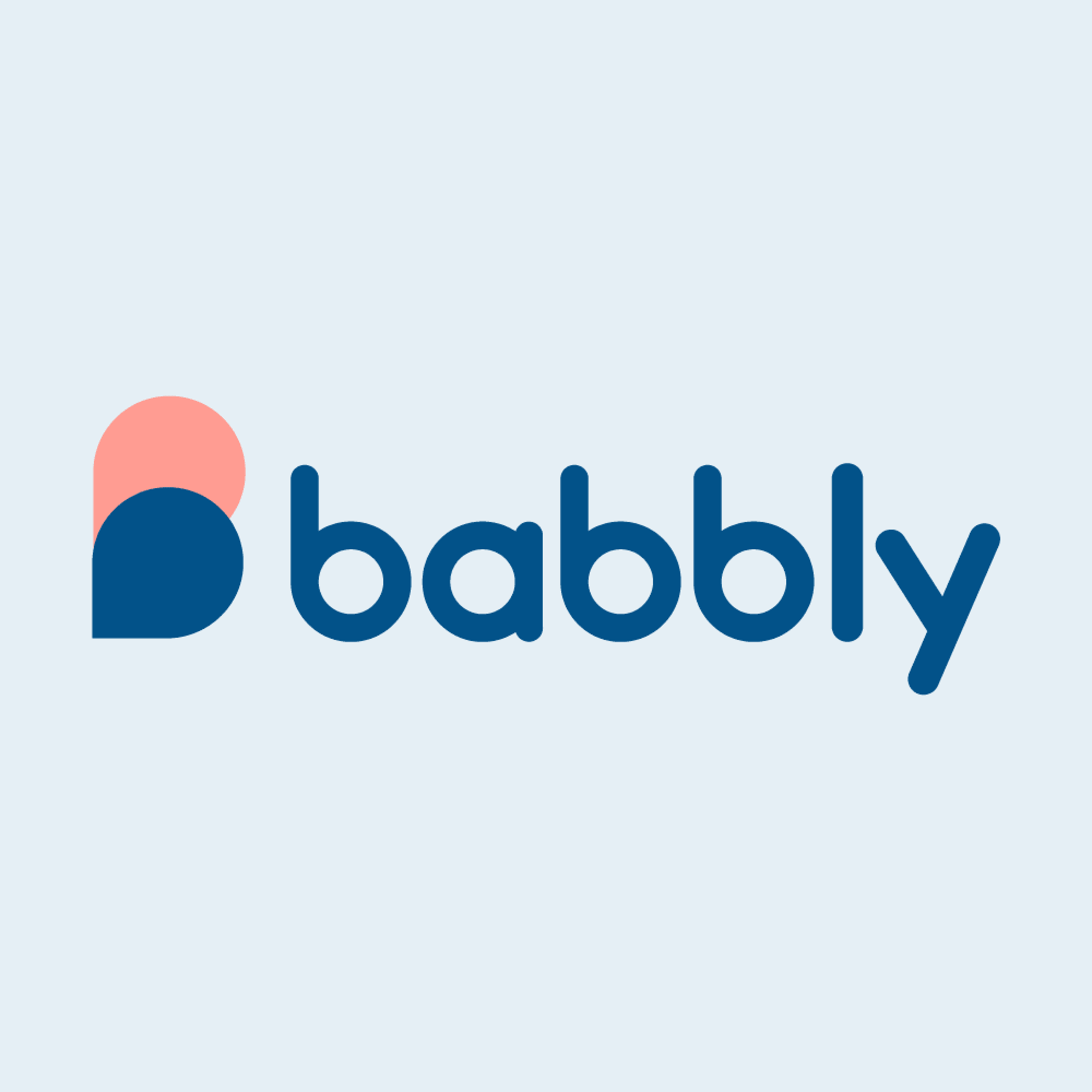
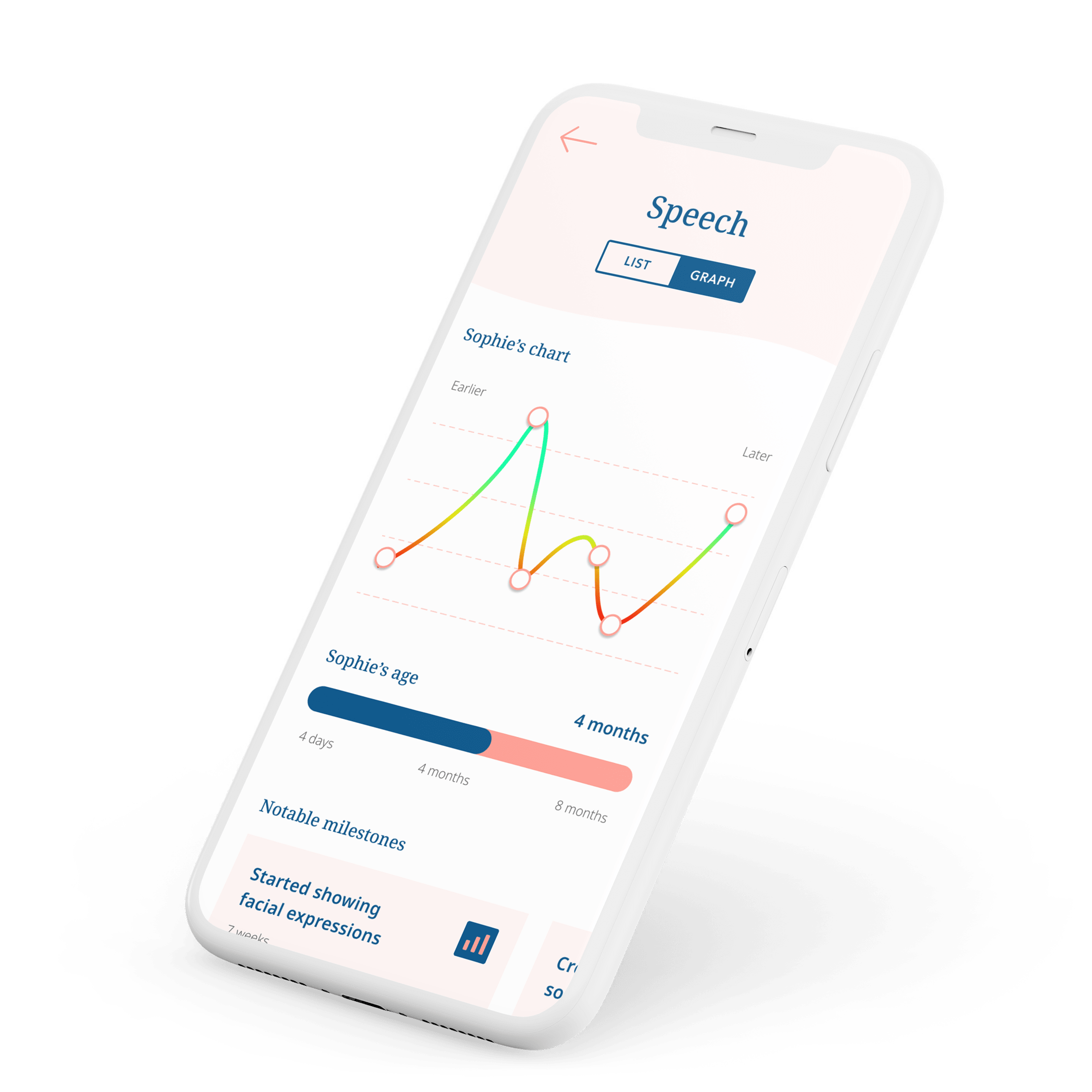
Babbly
An AI-enabled demo from concept to functional model in just 2 weeks
Babbly team closed a successful pre-seed investment round with machine learning PoC.
New Product Development & Artificial Intelligence
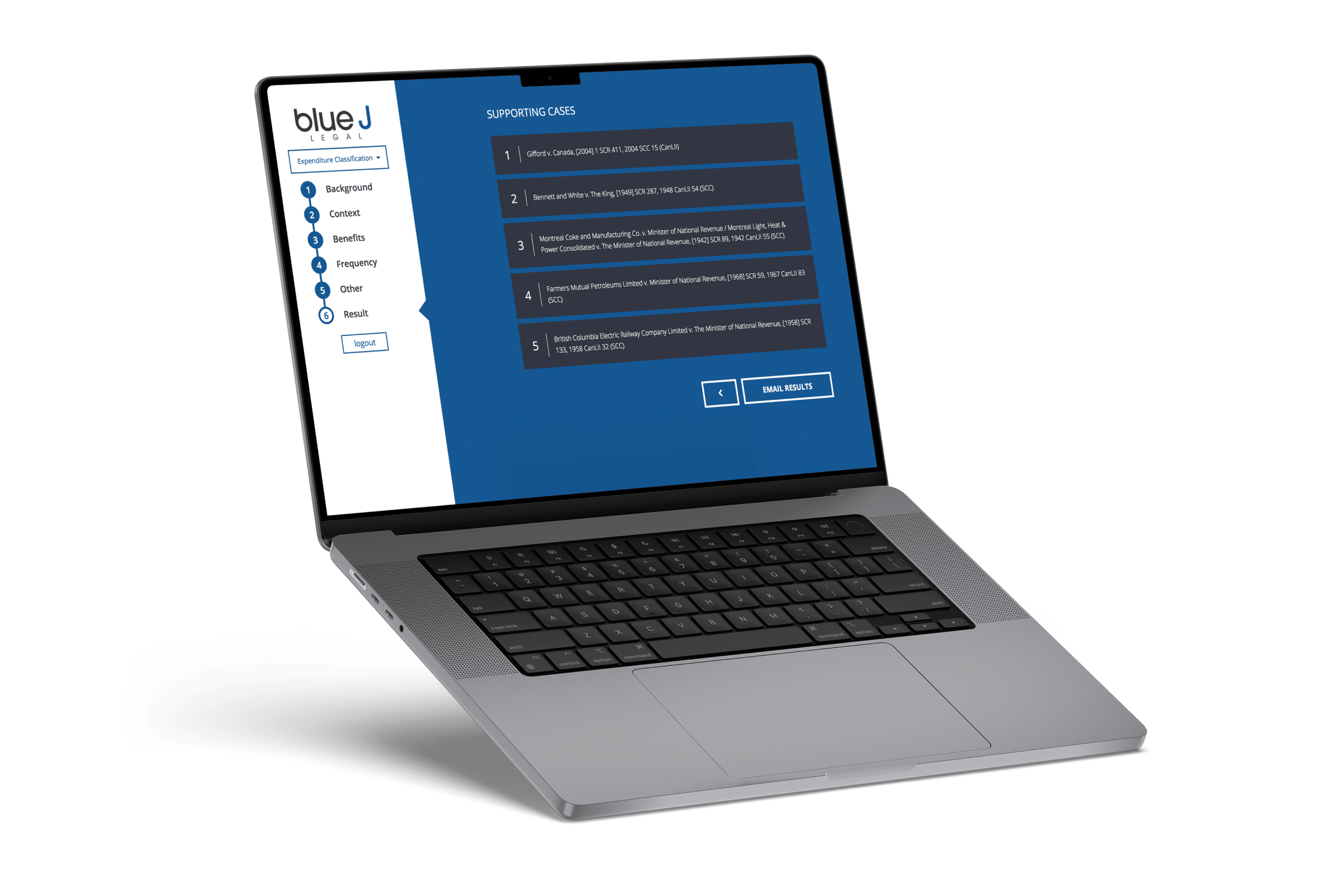
Blue J Legal
Simplifying the work of tax professionals with a mobile app
Blue J Legal successfully launched its AI-powered research tool and acquired its first paying customers during its partnership with Rangle.
Featured Posts
In this webinar, we talk about useful frameworks for companies thinking about deploying AI in their context and building products with it.
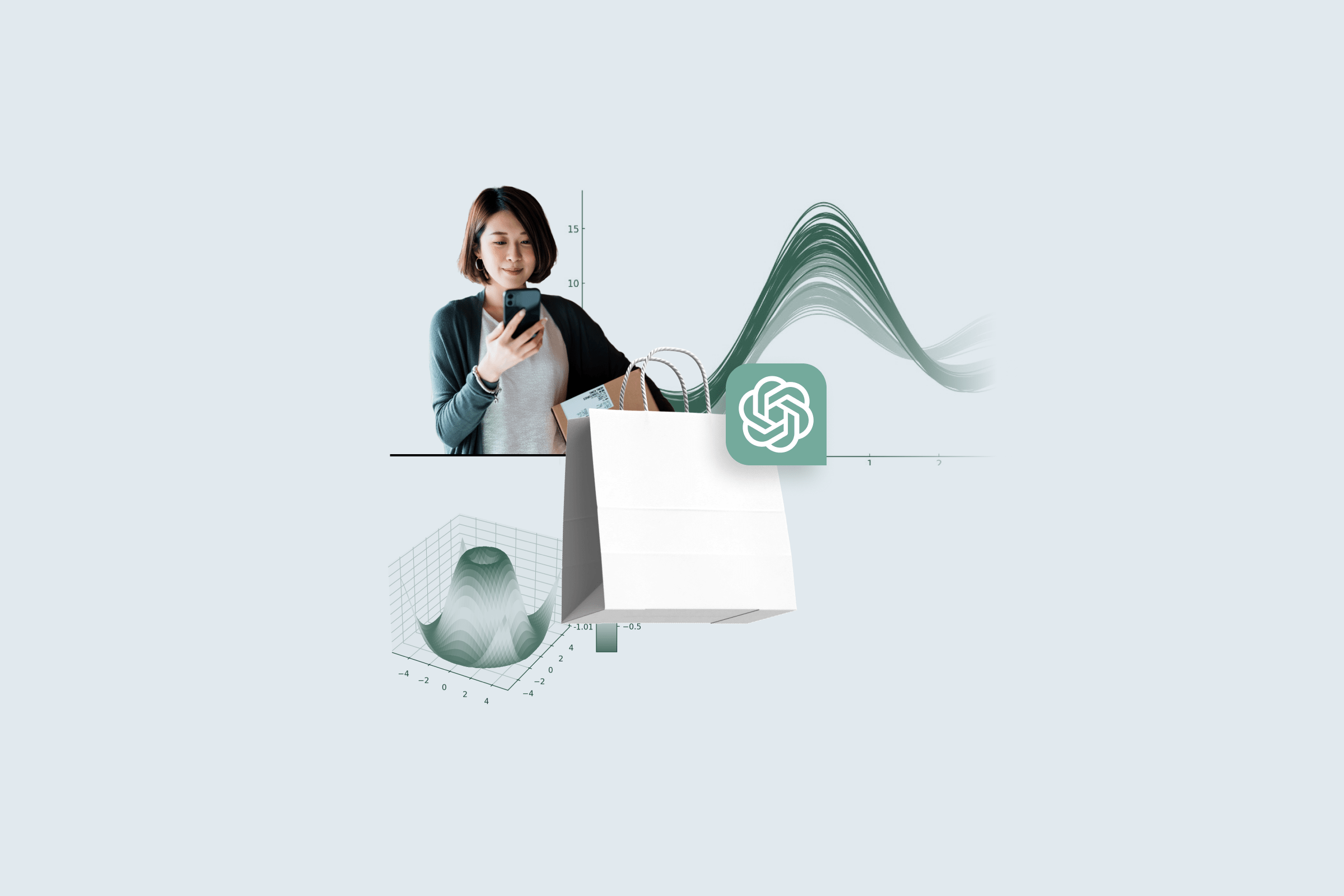
A practical guide to using AI to curate product collections at scale, make product recommendation and search algorithms smarter with semantic awareness, and empower teams to deliver seamless customer experiences.
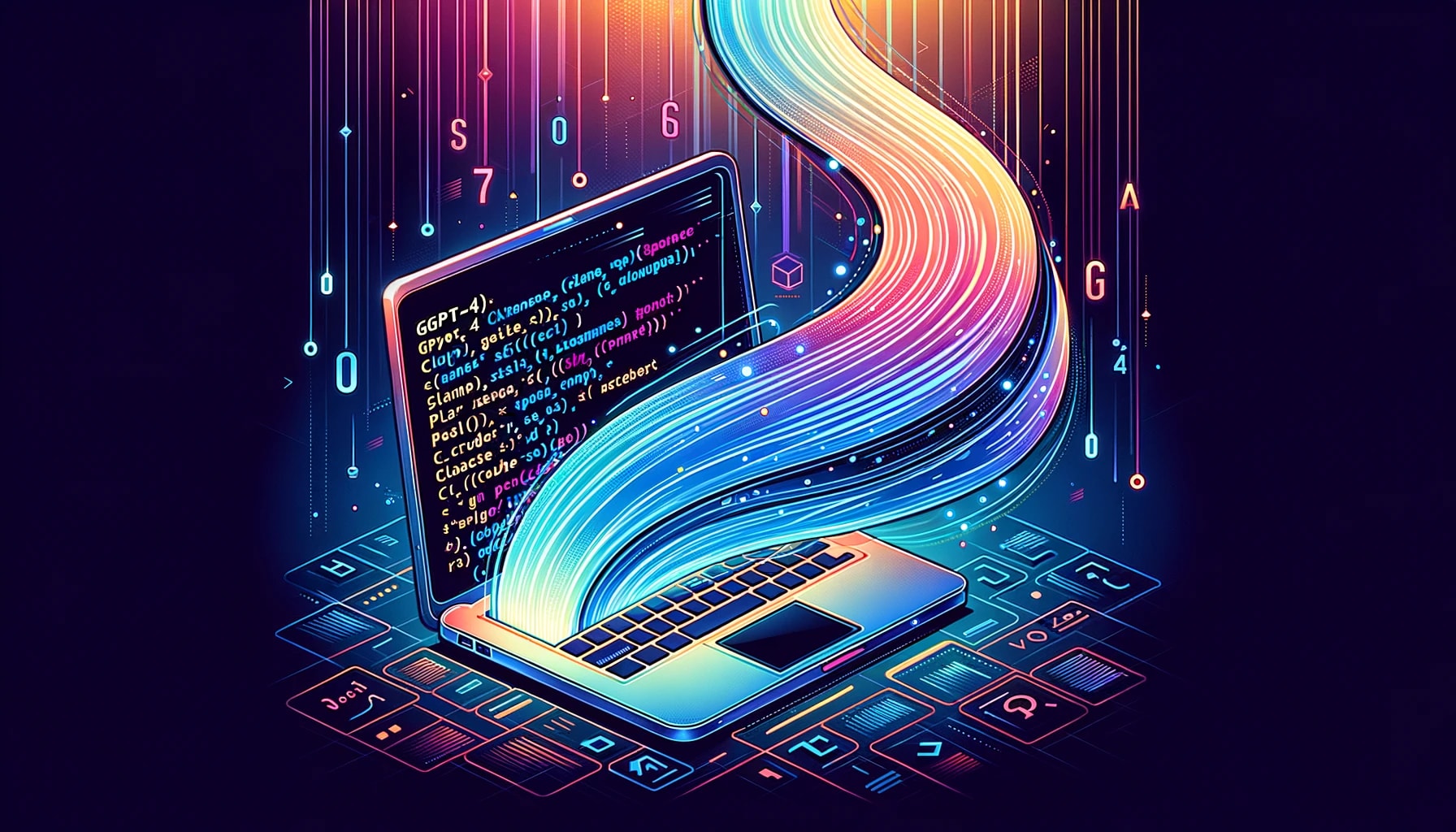
Generate nuanced translations for your e-commerce site using AI. Learn how to set up, fine-tune, and integrate AI models seamlessly with your headless CMS for automatic content translation. Uncover practical steps for implementation, from choosing the right language model to architecting efficient translation workflows that align with your budget and goals.